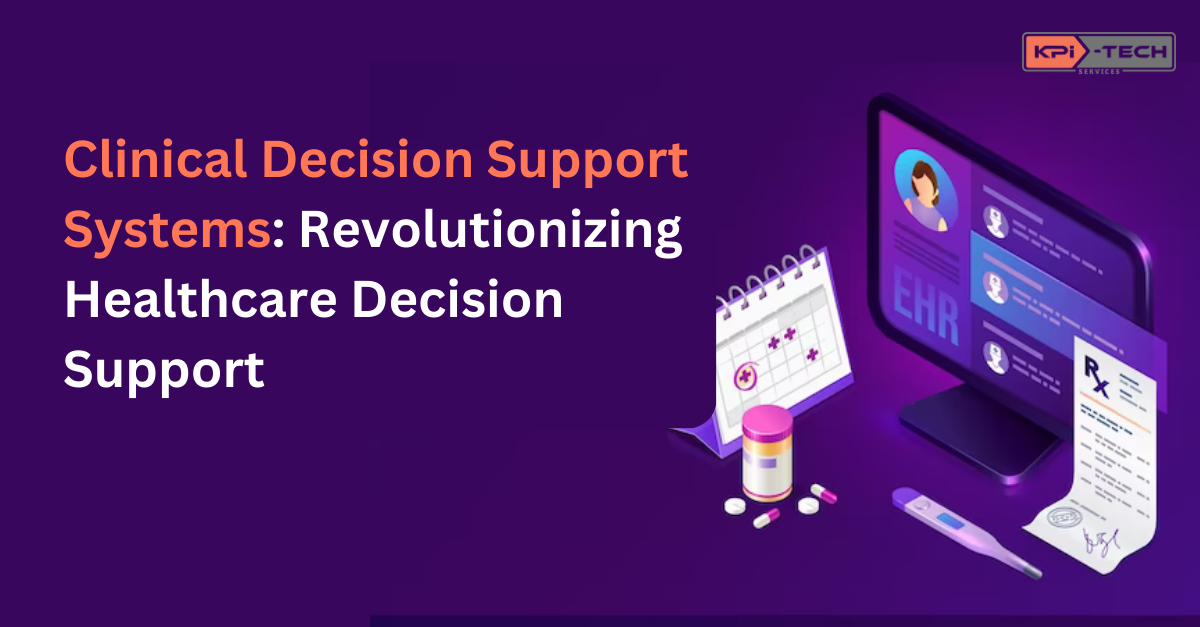
Clinical Decision Support Systems: Revolutionizing Healthcare Decision Support
Among the array of healthcare technologies, the clinical decision support system (CDSS) stands out as a game-changer, offering invaluable assistance to healthcare professionals in their intricate decision-making processes while lightening their cognitive load.
Experiencing an impressive compound annual growth rate of 10.5% from 2022 to 2030, clinical decision support systems are evolving at an unparalleled pace. Nonetheless, there remain concerns and constraints tied to CDSS, particularly regarding how their intricate algorithms translate into practical use.
This article provides an overview of decision support systems in the realm of healthcare, their applications, and the challenges they entail. It also draws a comparison between CDSS and Remote Patient Monitoring (RPM), illustrating how these technologies can complement each other. Upon reading, you will be better equipped to make an informed choice regarding the most suitable CDSS for your organization.
Key Functions of Clinical Decision Support Systems
Clinical Decision Support Systems (CDSS) are healthcare information technology systems designed to provide healthcare professionals with patient-specific recommendations to assist in clinical decision-making.
The primary functions of CDSS include:
- Drug Selection:This entails providing guidance on dosages, conducting checks for drug allergies, and analyzing potential drug interactions.
- Diagnostic Assistance: CDSS offers suggestions or advice on potential diagnoses based on patient data and symptoms, which can include diagnostic imaging.
- Clinical and Workflow Management: These systems help healthcare providers manage and streamline clinical processes and workflows. They may also send alerts and reminders to healthcare professionals, particularly regarding patients not adhering to their prescribed treatments.
- Order Entry: CDSS ensures the accuracy and safety of patient test orders, verifying the appropriateness of orders to reduce the risk of errors.
- Predictive Analytics: By analyzing extensive patient data, CDSS can predict potential health risks or outcomes, enabling proactive healthcare decision-making.
Types of clinical decision support systems
- Types of clinical decision support systems While there are various ways to classify clinical decision support systems (CDSS), they can generally be grouped into two fundamental categories: knowledge-based CDSS and non-knowledge-based CDSS.
- Knowledge-based CDSS possess a knowledge base and rely on existing data repositories to analyze and generate solutions. In contrast, non-knowledge-based CDSS employ machine learning techniques to analyze clinical data. They observe patterns in how routine tasks are performed and learn from these patterns.
- Knowledge-based clinical decision support applications are most suitable for situations where well-established medical guidelines exist. They are also highly effective in identifying and assessing drug interactions.
- Non-knowledge-based decision support solutions excel in scenarios requiring complex data analysis and predictive modeling, especially when explicit knowledge is limited. Some applications include image interpretation and providing personalized treatment recommendations.
The architecture of a clinical decision support system
The basic architecture of a clinical decision support system in healthcare consists of three main elements:
- Database/data management:The place where all relevant information is stored. This includes patient information, medical knowledge, clinical practice guidelines, and protocols.
- Interface engine:The system component that analyzes the information and makes decisions.
- User interface: This is the part of the system that the users (healthcare providers) interact with. It includes different features, including alerts and reminders.
Applications of CDSS
Clinical Decision Support Systems (CDSS) offer a wide range of applications depending on the specific software in use. Here, we explore some of the most common and crucial ones:
-
Reminders and Alerts
CDSS generates alerts related to drug interactions, health maintenance reminders, and recommendations for diagnostic tests. These alerts also notify healthcare professionals about critical laboratory test results and potentially risky patient statistics. The implementation of alerts plays a vital role in preventing medical errors.
-
Clinical Guidelines and Protocols
CDSS provides healthcare practitioners with guidelines for disease management and preventive care. These guidelines are derived from the latest research, best practices, and relevant medical protocols. CDSS contributes to increased adherence to these guidelines, enhancing the quality of care.
-
Order Entry and Documentation
Decision support solutions are seamlessly integrated with Electronic Health Records (EHR) and Computerized Provider Order Entry (CPOE) systems. This integration allows healthcare professionals to enter medication orders and various other patient care orders. The software evaluates these orders, identifying potential issues and suggesting alternatives, when necessary, thus promoting safe and effective healthcare delivery.
-
Patient Education
CDSS aids in patient education by generating personalized educational materials based on the individual patient's condition and prescribed medications.
-
Diagnostic Support
In addition to the mentioned applications, CDSS can also assist in diagnostic processes. It can offer recommendations and guidance to healthcare professionals when making diagnoses based on patient data and symptoms.
-
Predictive Analytics
CDSS leverages predictive analytics to analyze patient data and predict potential health risks or outcomes. This predictive capability enables proactive healthcare decision-making and interventions.
Top Clinical Decision Support Systems in 2023
In the rapidly evolving landscape of healthcare technology, Clinical Decision Support Systems (CDSS) are gaining prominence for their ability to assist healthcare professionals in making informed decisions and improving patient care. Here, we present an overview of some of the best CDSS available in 2023.
IBM Watson Health
- Micromedex Clinical Knowledge: A flagship CDSS used by over 4,500 medical establishments. It includes medication management tools, disease and condition management modules, and toxicology management for identifying poisons.
- Watson for Oncology: Utilizes AI to recommend cancer treatments after analyzing vast amounts of medical data.
- Watson for Genomics: Analyzes genetic data to provide personalized treatment options, with a focus on cancer genomics.
- Watson Care Manager: Assists in care management and creates individualized care plans.
- UpToDate by Wolters Kluwer Health: Covers a wide range of medical conditions, offering comprehensive information on diagnosis, management, and treatment options, integrated with most EHR systems
Siemens Healthineers
- Siemens' solutions emphasize reporting capacity and user-friendly AI, primarily compatible with Protis Data Management System and Siemens hardware.
- Healthineers: Often tied to diagnostics, with a focus on conditions where imaging or lab diagnostics play a pivotal role.
- AI-Rad Companion: A cloud-based AI decision support module for radiology, automating post-processing of imaging datasets.
Hearst (Zynx Health)
- A subsidiary of Hearst, Zynx Health offers evidence-based guidance for various conditions, with a primary focus on ensuring best-practice care.
- ZynxOrder: Provides evidence-based order sets for deployment and customization to decrease variability and improve patient outcomes.
- ZynxCare: Offers care plans based on best practices for nursing and interdisciplinary teams.
- ZynxAmbulatoryCare: An evidence-based decision support software for outpatient settings.
Cerner Clinical Decision Support
- Cerner's EHR system integrates clinical decision support across a wide range of conditions.
- Specialized tools for critical conditions, including sepsis detection and acute kidney injury prevention.
- Integration with Cerner's EHR systems, widely used worldwide.
Philips
- Philips' CDSS tools are designed for conditions requiring constant monitoring or involving significant diagnostic imaging.
- Integration with Siemens IntelliVue monitors.
- Solutions include IntelliSpace Cognition for assessing cognitive impairments, Horizon Trends for vital sign monitoring, and more.
Walter Kluwer
- Walter Kluwer provides comprehensive decision support solutions, including drug information and interaction checking, and real-time clinical surveillance and analytics tools.
These leading CDSS providers offer diverse solutions to meet the evolving needs of healthcare organizations, enhancing decision-making, patient care, and outcomes.
Challenges and limitations of CDSS
Despite obvious benefits of clinical decision support systems in healthcare, they also face limitations. Most of the limitations stem from the complexity of these systems.Healthcare IT companies can help your organization navigate the challenges. Here are a couple of the most significant ones:
- The algorithm transparency and trust:In clinical decision support solutions, the exact way decisions or predictions are made isn't always clear. While the system might generate a recommendation, sometimes the logic behind the predictions is hard to attain. If clinicians don't understand how the CDSS arrived at a particular decision, they are likely to not trust or act on its recommendations. Therefore, having transparency, proof of validation, and options for feedback is essential.
- Alert fatigue:One key function of CDSS is to provide alerts to healthcare providers. However, too many alerts, sometimes incorrect, can lead to 'alert fatigue.' This is a state when providers become desensitized and may start to ignore or override alerts, even critical ones. The system of priority alerts and alert customization can help address these problems.
- Maintenance of knowledge base:In healthcare, regulations and clinical guidelines are subject to constant change. Many types of clinical decision support systems are not that fast at adjusting to fast-paced changes. Timely maintenance and updates are an answer, but these can be expensive.
- Cost of implementation: About 74% of users report issues with the financial viability of CDSS in healthcare (Source). Initial implementation and customization are expensive, but maintenance and the need to train new staff add to the cost. Choosing modular solutions that accommodate your budget can solve this issue.
- Lack of interoperability:CDSS have significant interoperability challenges when integrating with EHR and other systems used in hospital settings. CDSS may be designed as isolated systems that can't communicate effectively with others. Issues also arise from programming complexities and the variety of clinical data sources. However, interoperability standards, such as HL7 and FHIR , can help solve these issues.
ConclusionÂ
Due to the complexity of CDSS development and integration, a good strategy is to involve a remote IT team in the development of decision support software.
Our team of experts can help you build custom CDSS from scratch or assist with adopting an existing CDSS in healthcare.
Here is what we can do:
- Ensure that the CDSS seamlessly integrates with existing Electronic Health Records (EHR) systems.
- Routinely update the CDSS with the latest guidelines and evidence-based practices.
- Monitor the system's performance.
- Set up mechanisms to collect user feedback and make iterative improvements to the CDSS based on this feedback.