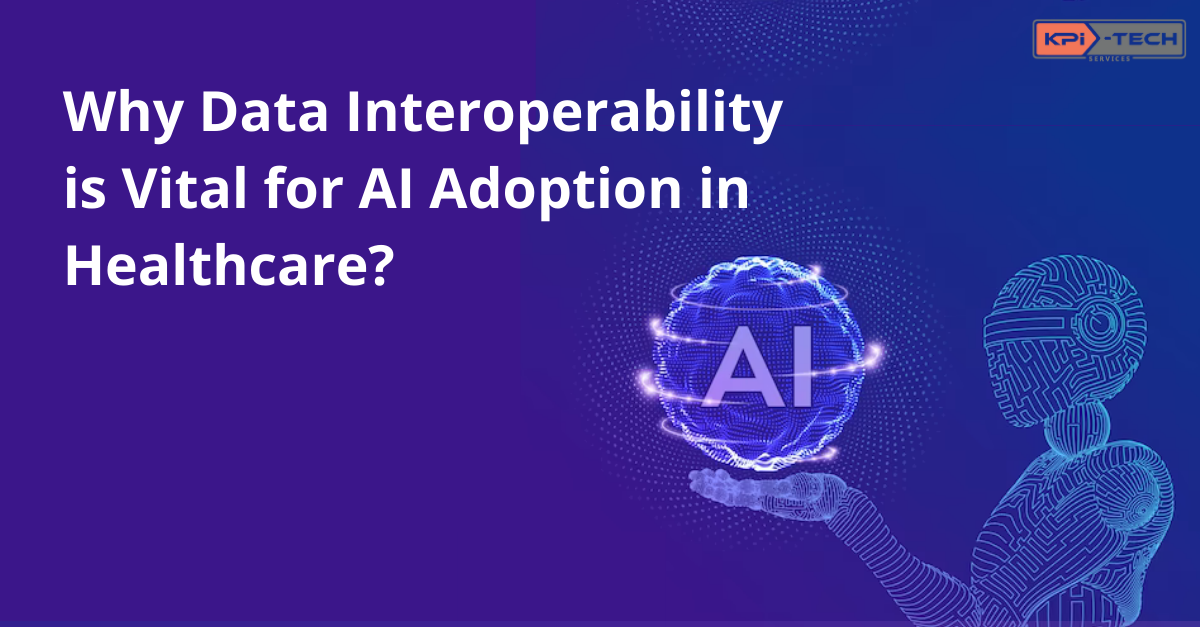
Why is Data Interoperability Vital for AI Adoption in Healthcare?
As the healthcare industry undergoes rapid digital transformation, the use of technologies like artificial intelligence (AI) is expected to have a significant influence on improving efficiency, quality, and scalability of healthcare outcomes. AI is already proving its worth by enhancing patient care through predictive capabilities, uncovering insights beyond human capabilities, and providing valuable context. The data generated by AI holds the potential to enable early disease detection, personalized treatments and medications, prevention of diagnostic or prescription errors, analysis of treatment risks, and much more. Clinical AI algorithms are already making strides in fields such as dermatology and radiology for image-based diagnoses, patient monitoring and management, genome interpretation, and drug discovery.
The Opportunity for AI Adoption in Healthcare
Despite the promising potential of artificial intelligence (AI) to enhance healthcare and drive better health outcomes, its adoption in the industry has been slow, as indicated by a report from the Brookings Institution on March 9. However, a recent survey conducted by health insurer Optum in December 2021 revealed that 85 percent of healthcare executives have developed an AI strategy, and nearly half of the executives surveyed are currently leveraging AI technology.
This indicates a unique opportunity for healthcare and life science organizations to leverage AI for faster, safer, and more impactful outcomes. However, in order to succeed, organizations must address crucial issues that currently hinder adoption and limit the potential impact of AI. These challenges include limitations on data storage, issues related to data access, and concerns surrounding data security and protection.
Related: How can Healthcare IT and Interoperability Companies empower Healthcare IT vendors?
AI Healthcare Use Cases in 2023
Medical Imaging Analysis
AI can analyze medical images such as X-rays, CT scans, and MRIs to detect and diagnose conditions like tumors, fractures, and abnormalities. It can help radiologists identify patterns and make more accurate assessments, leading to faster and more effective diagnoses.
Predictive Analytics
AI algorithms can analyze patient data, including medical history, lab results, and vital signs, to predict the likelihood of diseases or adverse events. This enables early intervention and personalized treatment plans.
Drug Discovery and Development
AI can speed up drug discovery by analyzing vast amounts of biological and chemical data to identify potential drug candidates. It can also optimize clinical trials and predict drug responses in specific patient populations. According to Deep Pharma Intelligence, investments in AI-driven drug discovery companies have experienced a significant surge, tripling over the past four years and reaching an impressive $24.6 billion in 2022.
Virtual Assistants and Chatbots
AI-powered virtual assistants can provide personalized health advice, answer patient queries, and assist in appointment scheduling. Chatbots can triage patients, provide basic medical information, and guide individuals to appropriate healthcare resources. Additionally, virtual nurses play a role in educating patients on proper medication usage. Data reveals that 75% of patients opt to interact with a virtual nurse rather than a human counterpart for such guidance
Remote Patient Monitoring
AI-enabled wearables and IoT devices can continuously monitor patients' vital signs, activity levels, and medication adherence. AI algorithms analyze the collected data to identify trends, detect anomalies, and alert healthcare providers in real-time.
Personalized Treatment Planning
AI algorithms can analyze large datasets of patient records, treatment outcomes, and medical literature to recommend tailored treatment plans based on individual characteristics. This approach can improve treatment efficacy and reduce adverse effects.
Administrative Workflow Automation
AI can automate administrative tasks, such as patient registration, billing, and coding, reducing the burden on healthcare staff and streamlining operations.
Disease Prevention and Population Health
AI algorithms can analyze population-level data to identify disease patterns, predict outbreaks, and recommend targeted interventions. This information can inform public health strategies and preventive measures.
Mental Health Support
AI-powered chatbots and virtual therapists can provide mental health support, offering personalized counseling, monitoring mood changes, and detecting signs of distress.
Artificial intelligence in the field of medicine provides optimism that leveraging this technology can potentially lead to rapid advancements in finding treatments for currently incurable diseases
Related: Unlocking Seamless Healthcare: How AI and Machine Learning Drive Interoperability.
Challenges to AI Adoption
As the demand for healthcare services continues to grow, healthcare providers are grappling with shortages of staff in various regions, which further strains the already burdened healthcare professionals. Moreover, patients' expectations of healthcare are evolving, as they actively participate in managing their own health and seek more accessible, convenient, and personalized care. Although there has been considerable research and innovation in the use of artificial intelligence (AI) in healthcare, obstacles to its clinical implementation persist. Challenges such as constraints on data storage, difficulties in seamless data access, and concerns regarding data security and privacy hinder the widespread adoption and potential impact of AI in the healthcare industry.
Data access and Interoperability challenges
The effectiveness of any AI application heavily relies on the data used to train its model. In the case of the U.S. healthcare system, which holds great potential for AI applications, there is a lack of comprehensive, representative, and standardized real-world health data that can be readily shared and accessed.
Collecting healthcare data is challenging, often disrupting the workflow of healthcare professionals who may view the process unfavorably, and the collected data tends to be incomplete. Additionally, pooling data from multiple hospitals or healthcare providers poses difficulties in terms of integration and collaboration. The lack of interoperability is a significant barrier to AI adoption in healthcare due to the following reasons:
Fragmented Data Sources
Healthcare data is often stored in various systems and formats across different institutions, making it difficult to access and integrate for AI applications.Electronic health records(EHRs) from different vendors may not be compatible, hindering the seamless exchange and aggregation of patient information.
Data Quality and Standardization
Inconsistent data quality and lack of standardized formats pose challenges for AI algorithms. Variations in data collection methods, coding systems, and terminology make it difficult to train and deploy AI models effectively across different healthcare settings.
Privacy and Security Concerns
Healthcare data is highly sensitive, and ensuring patient privacy and data security is paramount. Interoperability challenges add complexity to maintaining data confidentiality and complying with regulations, such as the Health Insurance Portability and Accountability Act (HIPAA).
Technical Hurdles
Integrating disparate data systems requires technical expertise, resources, and infrastructure. Legacy systems that lack modern interoperability standards and APIs may pose additional challenges for seamless data exchange and integration with AI platforms.
Governance and Legal Frameworks
Establishing governance models and legal frameworks to enable secure data sharing and protect patient rights across institutions is crucial. Resolving legal and policy issues related to data ownership, consent, and liability is necessary to encourage data sharing for AI initiatives.
Stakeholder Collaboration
Achieving interoperability requires collaboration among various stakeholders, including healthcare providers, technology vendors, policymakers, and regulatory bodies. Aligning interests, addressing concerns, and establishing common standards and protocols are essential for successful interoperability implementation
RELATED: Unlocking Seamless Healthcare: How AI and Machine Learning Drive Interoperability
Data Interoperability is Important for AI Development
Addressing these interoperability challenges requires concerted efforts from the healthcare industry, technology developers, and policymakers. Use of standardized data formats like HL7-FHIR, complying with rules and regulations like ONC cures act and Healthcare Certifications, implementing secure and privacy-preserving data exchange mechanisms using HIE services, and establishing interoperability frameworks are crucial steps to accelerate AI adoption in healthcare.
Data Integration between various platforms plays a crucial role in enabling intelligent algorithms to develop a shared understanding and facilitates greater flexibility for AI and ML models from different vendors. This collaborative approach not only accelerates innovation but also drives advancements in the field of AI.
Major technology companies like Facebook and Microsoft introduced the Open Neural Network Exchange (ONNX) around five years ago, aiming to facilitate seamless transfers between AI frameworks and ML models.
This groundbreaking initiative allows developers to conduct research without being tied to a specific AI framework, empowering them to easily switch between different tools and combinations. For instance, they can combine technologies such as Microsoft's Cognitive Toolkit with Facebook's Caffe2 or PyTorch.
RELATED: FHIR Implementation for Interoperability Success in Healthcare
Conclusion
By enabling seamless data exchange healthcare organizations have an incredible opportunity to capitalize on the real benefits of AI and other emerging technologies. Without interoperability, the development of AI would be constrained and available to just big tech.
KPi-Tech brings over 20 years of experience in delivering exceptional Healthcare IT services and solutions specifically tailored for the US Healthcare industry. Our dedicated team specializes in providing top-notch integration and interoperability services, including HL7-FHIR integration development, HIE services, and seamless data access through advanced Interface engines. With our extensive expertise in the Healthcare domain, we are well-equipped to assist you in successfully implementing AI technologies into your existing systems. Contact our knowledgeable experts today to explore how we can elevate your healthcare organization's capabilities and drive innovation through interoperability and AI integration.